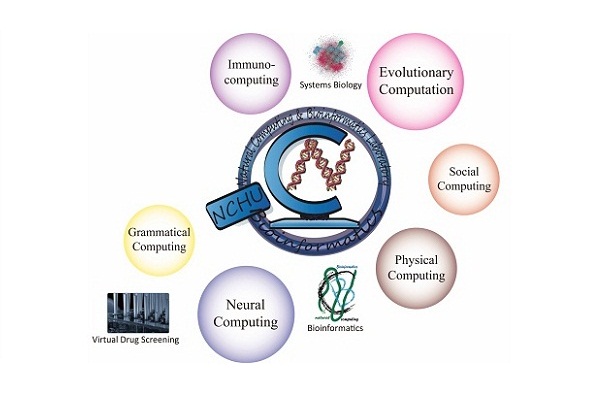
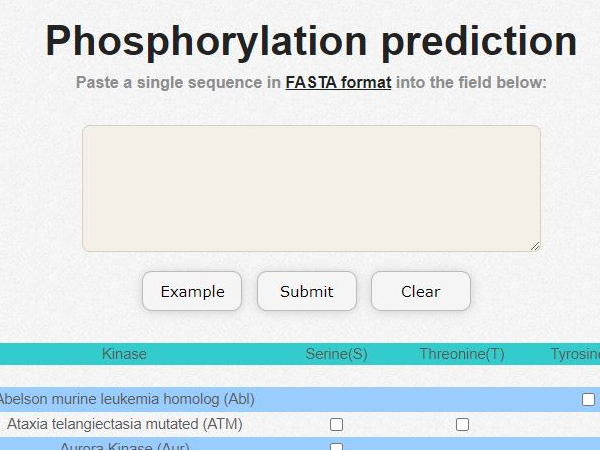
GasPhos
Protein phosphorylation is one of the important post-translational modifications, many biological processes are related with phosphorylation, such as DNA repair, transcriptional regulation and signal transduction. Therefore, abnormal regulations of phosphorylation usually cause diseases. If we can accurately predict human phosphorylation sites, this could help to solve human-related diseases. Therefore, this study developed a kinase-specific phosphorylation prediction system, GasPhos, and proposed a feature selection method, called Gas, based on ant colony system and genetic algorithm, and the performance evaluation strategy was used to choose the best learning model for different kinases. Gas uses MDGI as heuristic value on path selection, and adopted binary transition strategies and proposed a new transition rules. GasPhos can predict phosphorylation sites for 20 kinases; however, this article is focuses on six kinases with the properties of larger and common. By 5-foldcross-validation, the average performance of GasPhos is higher than the other five phosphorylation prediction system 10% of Matthews’s correlation coefficient (MCC). In system analysis, we discussed different heuristic value, the role of GA, three kinds of transformation rules, different feature selection methods and the biological properties that frequently selected features; in addition, we observed the correlation of Weblogo and the selected feature number of Gas. In order to let users more precisely using GasPhos, we analyzed the performance of each prediction system for different functional proteins and explored two kinds of human disease-related phosphorylation.
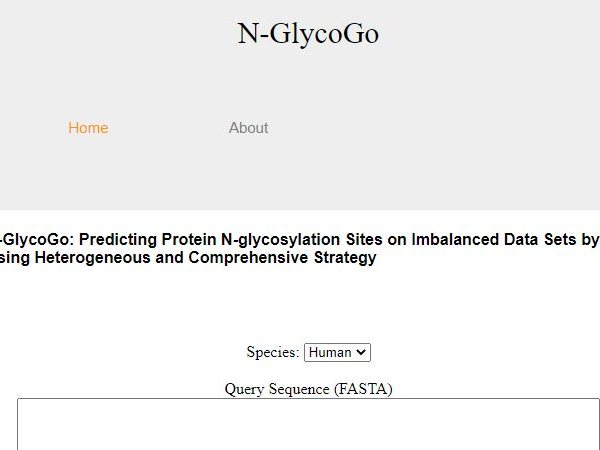
N-GlycoGo
Glycosylation is the most complex post-modification effect of proteins. It participates in many biological processes in the human body and is closely related to many disease states. Among them, N-linked glycosylation is the most contained glycosylation data. However, the current N-linked glycosylation prediction tool does not take into account the serious imbalance between positive and negative data. In this study, a comprehensive strategy was used to construct the N-linked glycosylation prediction model N-GlycoGo. And through protein sequence fragments and amino acid characteristics, a total of 11 heterogeneous features are encoded based on sequence, structure and function. And choose the best XGBoost integrated model as modeling. Finally, in the independent tests of human and mouse prediction models, N-GlycoGo is also superior to other tools. The MCC is 0.397 and 0.719, respectively, which is higher than the currently published glycosylation point prediction tools. We have successfully developed a fast and accurate N-linked glycosylation site prediction tool N-GlycoGo.
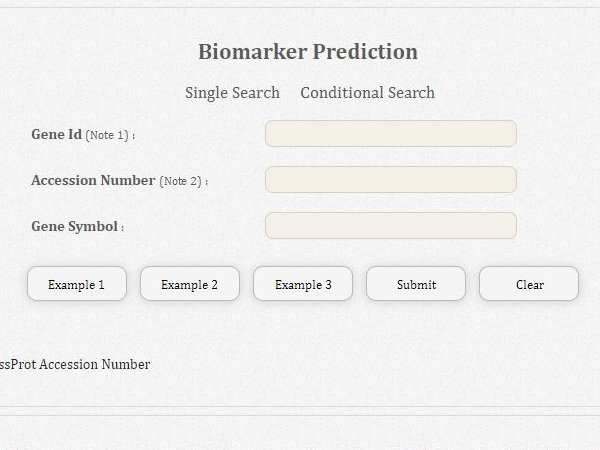
dBMHCC
Hepatocellular carcinoma (HCC), which is associated with an absence of obvious symptoms and poor prognosis, is the second leading cause of cancer death worldwide. Genome-wide molecular biology studies should provide biological insights into HCC development. Based on the importance of phosphorylation for signal transduction, several protein kinase inhibitors have been developed that improve the survival of cancer patients. However, a comprehensive database of HCC-related phosphorylated biomarkers and prediction platform has been lacking. We have thus curated the HCC-611, GOCU, PhosMotif, SPPKinase, MKA, and Drug databases to provide expression profiles, phosphorylation and drug information, and evidence type; gathered information on HCC-related pathways and their involved genes as candidate HCC biomarkers; and established a system for evaluating protein phosphorylation and HCC-related biomarkers to improve the reliability of biomarker prediction. The resulting dBMHCC contains 611 notable HCC-related genes, 234 HCC-related pathways, 17 phosphorylation-related motifs and their 255 corresponding protein kinases, 5955 HCC biomarkers, and 1077 predicted HCC phosphorylated biomarkers (HCCPMs). dBMHCC is an open resource for HCC phosphorylated biomarkers, which provides expression profiles, evidence type, and drug information to support researchers investigating the development of HCC and designing novel diagnosis methods and drug treatments.
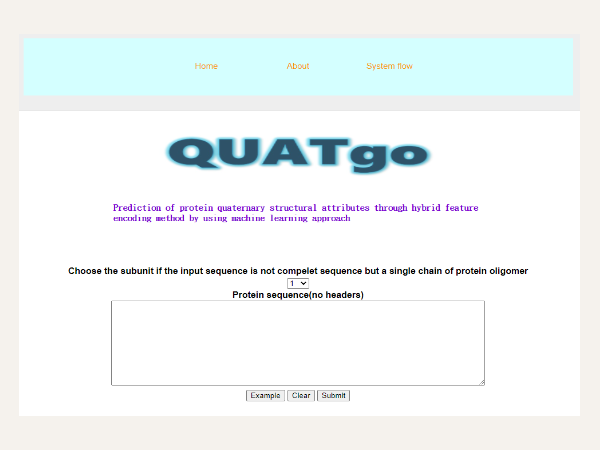
QUATgo
Many protein exist in natures as oligomers with various different quaternary structural attributes rather than a single chain. Predicting their attributes is an essential task in computational biology for the advancement of the proteomics. However, the existing methods did not consider the integration of heterogeneous coding and the accuracy of subunit categories with low data number. To end this, we proposed a predictive tool which can predicting more than 12 subunit protein oligomers, QUATgo. At the same time, three kinds of sequence coding were used, including dipeptide composition which was first time using to predict protein quaternary structural attributes, protein half-life characteristics and we modified the coding method of the Functional Domain Composition which proposed by the predecessors to solve the problem of large feature vectors. QUATgo solves the problem of insufficient data in a single subunit using a two-stage architecture and uses 10 times cross-validation to test the predictive accuracy of the classifier, the first-stage prediction model uses a random forest algorithm to generate sixteen homologous, heterologous oligomers and monomer respectively. The accuracy of the first-stage classifier is 63.4%. However, the number of training data of the hetero-10mer is insufficient so the training data of the hetero-10mer and the hetero-more than 12mer is regarded as the same category X. If the result of the first stage classifier is class X the sequence will sent to second stage classifier which was constructed with support vector machines, and can the prediction result of the hetero-10mer and hetero-more than 12mer with an accuracy of 97.5%, QUATgo will eventually have 61.4% cross-validation accuracy and 63.4% independent test accuracy. In case study, QUATgo can accurately predicts the variable complex structure of the MERS-CoV ectodomains.
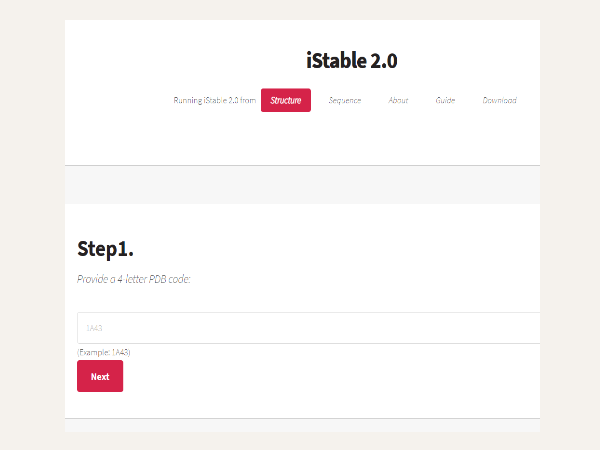
iStable2.0
A single mutation on the amino acid residue may cause a severe change in the whole protein structure and thus, lead to disruption of function. Predicting the protein stability changes can provide several possible candidates for the novel protein designing. We first proposed an integrated predictor, iStable, to solve the conflicting prediction results from different tools could cause confusion to users (1). In this web server issue, a new web tool, iStable 2.0, was constructed for three purposes. 1) To construct stand-alone program module for reducing the risk of broken of sub prediction web servers. 2) To add in sequence coding and XGBoost for improving the prediction accuracy. 3) To visualize four kinds of output data for protein designing. The flowchart of iStable 2.0 is as follows:
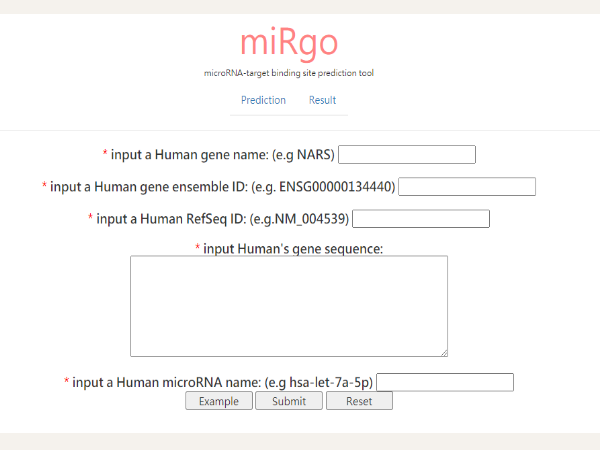
miRgo
MicroRNAs (miRNAs) are short non-coding RNAs that regulate gene expression and biological processes through binding to messenger RNAs. Predicting the relationship between miRNAs and their targets is crucial for research and clinical applications. Many tools have been developed to predict miRNA-target interactions, but variable results among the different prediction tools have caused confusion for users. To solve this problem, we developed miRgo, an application that integrates many of these tools. To train the prediction model, extreme values and median values from four different data combinations, which were obtained via an energy distribution function, were used to find the most representative dataset. Support vector machines were used to integrate 11 prediction tools, and numerous feature types used in these tools were classified into six categories-binding energy, scoring function, evolution evidence, binding type, sequence property, and structure-to simplify feature selection. In addition, a novel evaluation indicator, the Chu-Hsieh-Liang (CHL) index, was developed to improve the prediction power in positive data for feature selection. miRgo achieved better results than all other prediction tools in evaluation by an independent testing set and by its subset of functionally important genes.
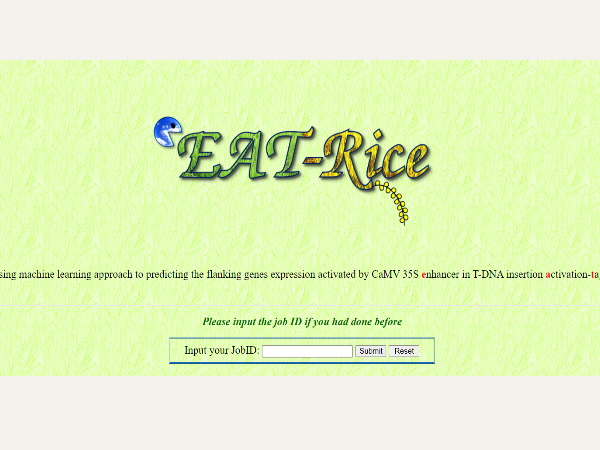
EAT-Rice
The rice T-DNA insertion mutants from TRIM database provide important genetic resources for gene function assay. Each T-DNA insertion mutant carried four tandem repeats of CaMV 35S enhancer which could activate the expression of its flanking genes and was known as the activation-tagged mutant. Previous studies showed the expression of flanking genes were neither uniformly affected by 35S enhancer nor correlated with their distance from the 35S enhancer.
Therefore, it will be a time-consuming and laborious works to check the expression of flanking genes when many genes were located near the T-DNA insertion site. In this study, we used machine learning approach to predict the expression of flanking genes in T-DNA activation-tagged mutants and used this analysis platform to further identify the function of candidate genes. The flanking genes, totally 358 genes that revealed either activated or no-activated in previous experimentally validated datasets were collected and three different regions of their DNA sequences including 1) Ups1k (one kb of upstream sequence from the start codon), 2) Distance (from the start codon of target gene to enhancer) and 3) Middle (150 bp of up- and downstream sequence around the central nucleotide of Distance region) were retrieved respectively to encode and build a two-layer machine learning prediction models.
In the first layer models, the features with sequences of permutations and combinations calculating by n-gram, specific motif on promoter, nucleotides physicochemical properties and CG-island were used to build SVM models by analyzed the hidden information of three sequences. Moreover, we adopted logistical regression to estimate probability of gene activated, it was depended for weighted of feature encoding. In the second layer models, the NaïveBayes Updateable algorithm was selected from 69 classified methods to integrate this first layer models, and the system performance was 88.33% on 5 fold cross-validation, and 79.17% on independent-testing finally. To evaluate the systemic accuracy with the distance of target genes from the enhancer, results showed the accuracy was much better for genes with distance distributed between 2 to 5 kb and 10 to 20 kb respectively; and further discussed the difference of gene activated information from TRIM. Taken together, this study successfully constructed a prediction system: EAT-Rice, which could provide the expression status of flanking genes in T-DNA insertion activation-tagged mutants.
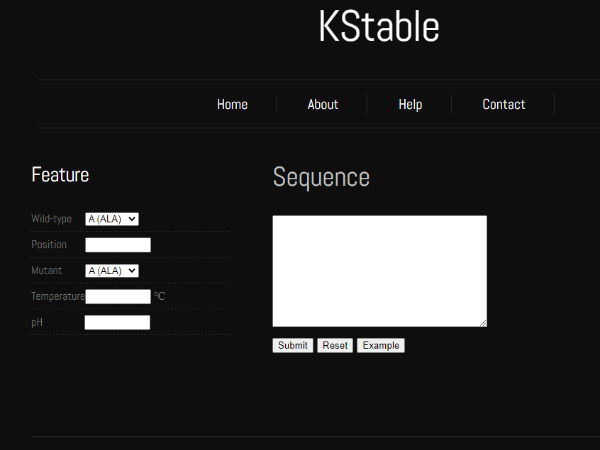
KStable
Protein thermostability is essential for both research and industrial applications. Up until now, most protein stability prediction tools considered 3D structure information. However, a large number of proteins possess only primary structure. Therefore, this study proposed an effective prediction system, KStable, based on protein sequence. KStable was the first to adopt KStar algorithms with regular-mRMR feature selection. The prediction accuracy of KStable was 0.83 on the Protherm database using 10-fold cross validation. In addition, we also compared KStable with present prediction tools (AUTO-MUTE, i-Mutant, Mupro, PopMuSiC and CUPSAT) and the prediction accuracy and the Matthew's correlation coefficient of KStable were better than others. Therefore, KStable was shown to reduce prediction time while keeping the prediction performance comparable to those that used 3D structure information.
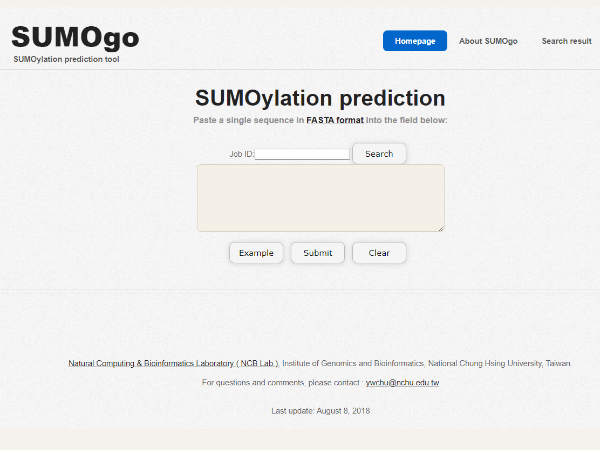
SUMOgo
Small Ubiquitin-like Modifier ( SUMO) proteins are a family of small proteins that are covalently attached to and detached from other proteins in cells to modify their function. Sumoylation is a post-translational modification involved in various cellular processes, such as gene expression, DNA repair, chromosome assembly, and cellular signaling.
Recently, most sumoylation prediction tools are using algorism, protein physicochemical and biochemical properties or consensus motif to predict modification sites. But those tools rarely mention to the effect of other post translational modification (PTM) on sumoylation prediction.
We develop a sumoylation prediction tool considered other PTM with support vector machine and name it SUMOgo.
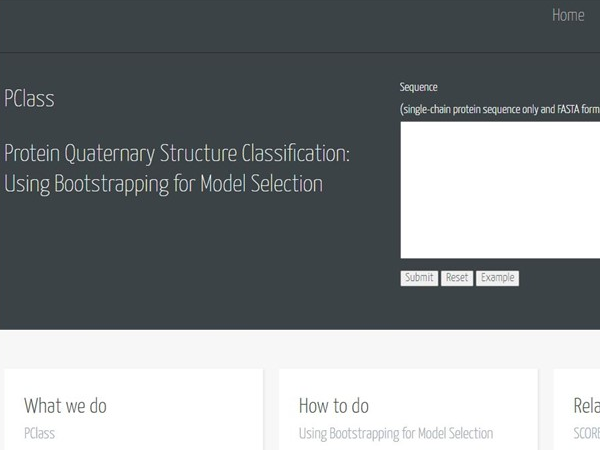
PClass
Protein quaternary structure complex is also known as a multimer, which plays an important role in a cell. The dimer structure of transcription factors is involved in gene regulation, but the trimer structure of virus-infection-associated glycoproteins is related to the human immunodeficiency virus. The classification of the protein quaternary structure complex for the post-genome era of proteomics research will be of great help. Classification systems among protein quaternary structures have not been widely developed. Therefore, we designed the architecture of a two-layer machine learning technique in this study, and developed the classification system PClass. The protein quaternary structure of the complex is divided into five categories, namely, monomer, dimer, trimer, tetramer, and other subunit classes. In the framework of the bootstrap method with a support vector machine, we propose a new model selection method. Each type of complex is classified based on sequences, entropy, and accessible surface area, thereby generating a plurality of feature modules. Subsequently, the optimal model of effectiveness is selected as each kind of complex feature module. In this stage, the optimal performance can reach as high as 70% of Matthews correlation coefficient (MCC). The second layer of construction combines the first-layer module to integrate mechanisms and the use of six machine learning methods to improve the prediction performance. This system can be improved over 10% in MCC. Finally, we analyzed the performance of our classification system using transcription factors in dimer structure and virus-infection-associated glycoprotein in trimer structure.
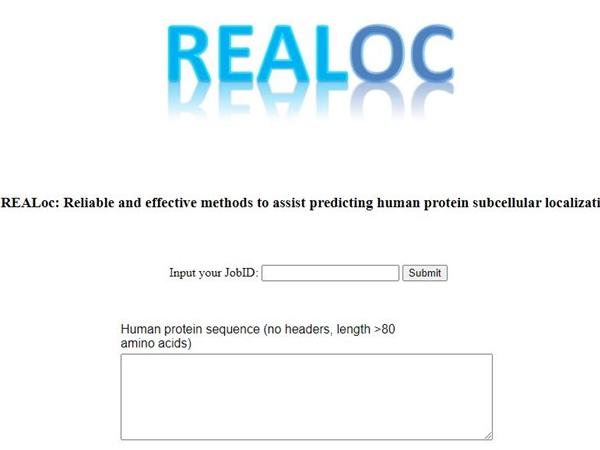
REALoc
Drug development and investigation of protein function both require an understanding of protein subcellular localization. We developed a system, REALoc, that can predict the subcellular localization of singleplex and multiplex proteins in humans. This system, based on comprehensive strategy, consists of two heterogeneous systematic frameworks that integrate one-to-one and many-to-many machine learning methods and use sequence-based features, including amino acid composition, surface accessibility, weighted sign aa index, and sequence similarity profile, as well as gene ontology function-based features. REALoc can be used to predict localization to six subcellular compartments (cell membrane, cytoplasm, endoplasmic reticulum/Golgi, mitochondrion, nucleus, and extracellular). REALoc yielded a 75.3% absolute true success rate during five-fold cross-validation and a 57.1% absolute true success rate in an independent database test, which was >10% higher than six other prediction systems. Lastly, we analyzed the effects of Vote and GANN models on singleplex and multiplex localization prediction efficacy.
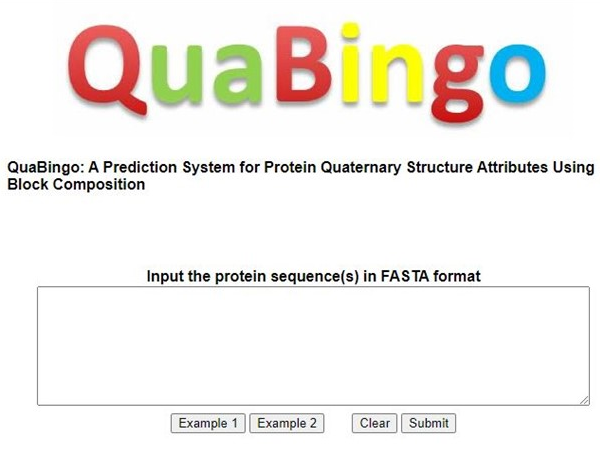
QuaBingo
Quaternary structures of proteins are closely relevant to gene regulation, signal transduction, and many other biological functions of proteins. In the current study, a new method based on protein-conserved motif composition in block format for feature extraction is proposed, which is termed block composition.
The protein quaternary assembly states prediction system which combines blocks with functional domain composition, called QuaBingo, is constructed by three layers of classifiers that can categorize quaternary structural attributes of monomer, homooligomer, and heterooligomer. The building of the first layer classifier uses support vector machines (SVM) based on blocks and functional domains of proteins, and the second layer SVM was utilized to process the outputs of the first layer. Finally, the result is determined by the Random Forest of the third layer. We compared the effectiveness of the combination of block composition, functional domain composition, and pseudoamino acid composition of the model. In the 11 kinds of functional protein families, QuaBingo is 23% of Matthews Correlation Coefficient (MCC) higher than the existing prediction system. The results also revealed the biological characterization of the top five block compositions.
QuaBingo provides better predictive ability for predicting the quaternary structural attributes of proteins.
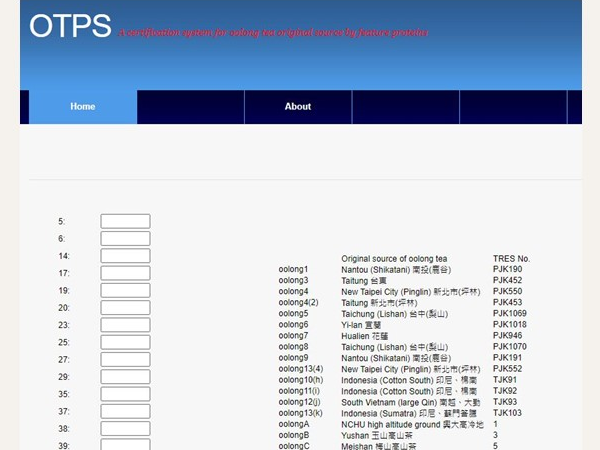
OTPS
Taiwan is known for its high quality oolong tea. Because of high consumer demand, some tea manufactures mix lower quality leaves with genuine Taiwan oolong tea in order to increase profits. Robust scientific methods are, therefore, needed to verify the origin and quality of tea leaves. In this study, we investigated whether two-dimensional gel electrophoresis (2-DE) and nanoscale liquid chromatography/tandem mass spectroscopy (nano-LC/MS/MS) coupled with a two-layer feature selection mechanism comprising information gain attribute evaluation (IGAE) and support vector machine feature selection (SVM-FS) are useful in identifying characteristic proteins that can be used as markers of the original source of oolong tea. Samples in this study included oolong tea leaves from 23 different sources. We found that our method had an accuracy of 95.5% in correctly identifying the origin of the leaves. Overall, our method is a novel approach for determining the origin of oolong tea leaves.
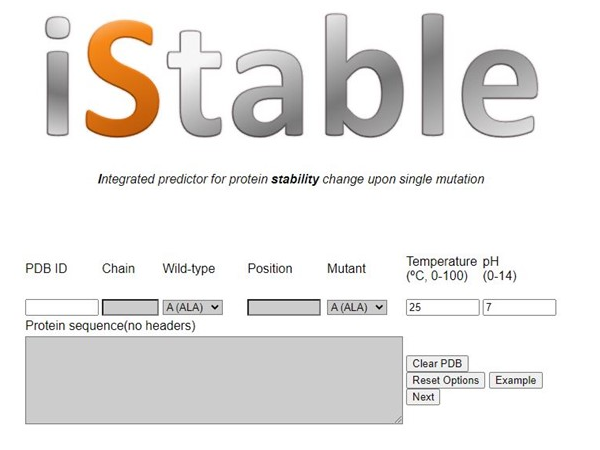
iStable
Mutation of a single amino acid residue can cause changes in a protein, which could then lead to a loss of protein function. Predicting the protein stability changes can provide several possible candidates for the novel protein designing. Although many prediction tools are available, the conflicting prediction results from different tools could cause confusion to users.
We proposed an integrated predictor, iStable, constructed by using sequence information and prediction results from different element predictors. In the learning model, iStable adopted the support vector machine as an integrator, while not just choosing the majority answer given by element predictors. Furthermore, the role of the sequence information played was analyzed in our model, and an 11-window size was determined. On the other hand, iStable is available with two different input types: structural and sequential. After training and cross-validation, iStable has better performance than all of the element predictors on several datasets. Under different classifications and conditions for validation, this study has also shown better overall performance in different types of secondary structures, relative solvent accessibility circumstances, and protein memberships in different superfamilies.
The trained and validated version of iStable provides an accurate approach for prediction of protein stability change.
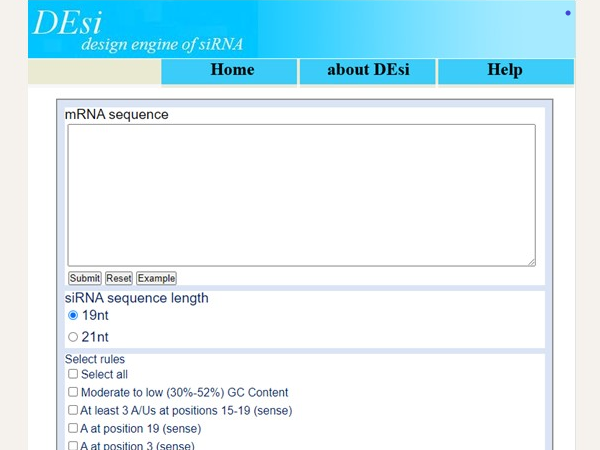
DEsi
Motivation: RNA interference (RNAi) by small interfering RNAs (siRNAs) has become a powerful tool in the fields of molecular biology and medicine. The success of RNAi gene silencing depends on the specificity of siRNAs for particular mRNA sequences. Some siRNA design guidelines have been established from siRNA sequence analysis, but designing siRNA sequences based only on these limited rules might not be effective. Experimentally validated siRNA databases have been developed over the past few years. Because of this wealth of sequence data, modules that employ machine learning methods can be developed to predict siRNA accuracy and optimize design.
Results: In this study, we created an siRNA design tool "DEsi" that quickly selects siRNAs with high RNAi activity against a desired mRNA. DEsi combines traditional feature filters, machine learning models and BLAST to optimize siRNAs design. The prediction models in DEsi had considerable predictive power, which was validated by statistical analysis. Compared with other siRNA design tools, DEsi can quickly and accurately design siRNAs against desired mRNAs.
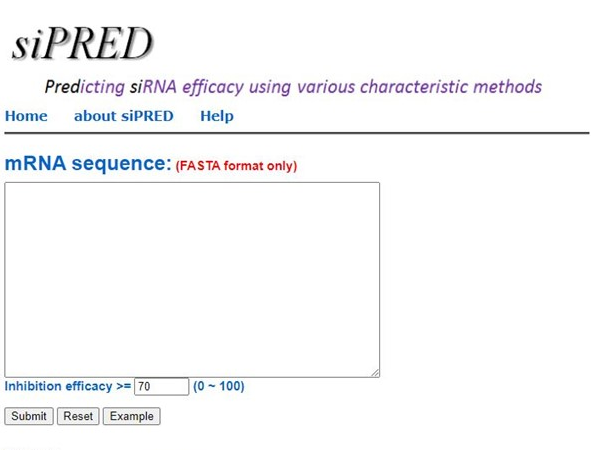
siPRED
Small interfering RNA (siRNA) has been used widely to induce gene silencing in cells. To predict the efficacy of an siRNA with respect to inhibition of its target mRNA, we developed a two layer system, siPRED, which is based on various characteristic methods in the first layer and fusion mechanisms in the second layer. Characteristic methods were constructed by support vector regression from three categories of characteristics, namely sequence, features, and rules. Fusion mechanisms considered combinations of characteristic methods in different categories and were implemented by support vector regression and neural networks to yield integrated methods. In siPRED, the prediction of siRNA efficacy through integrated methods was better than through any method that utilized only a single method. Moreover, the weighting of each characteristic method in the context of integrated methods was established by genetic algorithms so that the effect of each characteristic method could be revealed. Using a validation dataset, siPRED performed better than other predictive systems that used the scoring method, neural networks, or linear regression. Finally, siPRED can be improved to achieve a correlation coefficient of 0.777 when the threshold of the whole stacking energy is ≥ –34.6 kcal/mol.
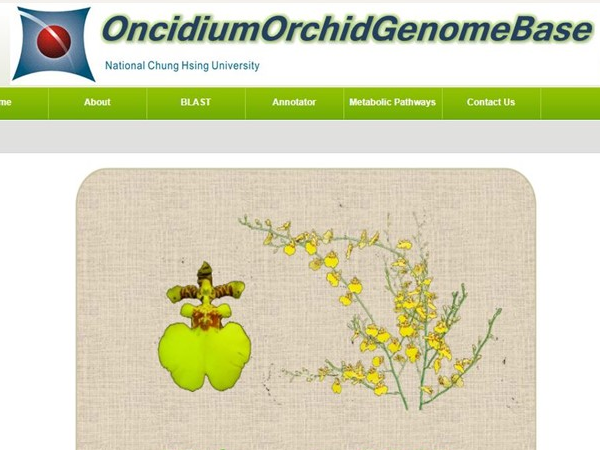
OncidiumOrchid GenomeBase
Oncidium 'Gower Ramsey' is a valuable and successful commercial orchid for the floriculture industry in Taiwan. However, no genome reference sequence currently exists for Oncidium orchids, to facilitate the development of molecular biological studies and the breeding of these orchids. In this study, we generated Oncidium cDNA libraries for six different organs, including leaves, pseudobulbs, young inflorescences, inflorescences, flower buds and mature flowers. We utilized 454-pyrosequencing technology to perform high-throughput deep sequencing of the Oncidium transcriptome, yielding more than 0.9 million reads with an average length of 328 bp, for a total of 301 million bases. De novo assembly of the sequences yielded 50,908 contig sequences with an average length of 493 bp from 796,463 reads and 120,219 singletons. The assembled sequences were annotated using BLAST, and a total of 12,757 and 13,931 UniGene transcripts from the Arabidopsis and rice genomes were matched by TBLASTX, respectively. A Gene Ontology (GO) analysis of the annotated Oncidium contigs revealed that the majority of sequenced genes were associated with cellular processes, unknown molecular functions and intracellular components. Furthermore, a complete flowering-associated expressed sequence that included most of the genes in photoperiod pathway and the 15 CONSTANS-LIKE (COL) homologs with the conserved CCT domain was obtained in this collection. These data revealed that the Oncidium EST database generated in this study has sufficient coverage to be used as a tool to investigate the flowering pathway and various other biological pathways in orchids.